Alright, so I’m gonna break down how I tackled those Miami vs. New York predictions. It was a wild ride, lemme tell ya.
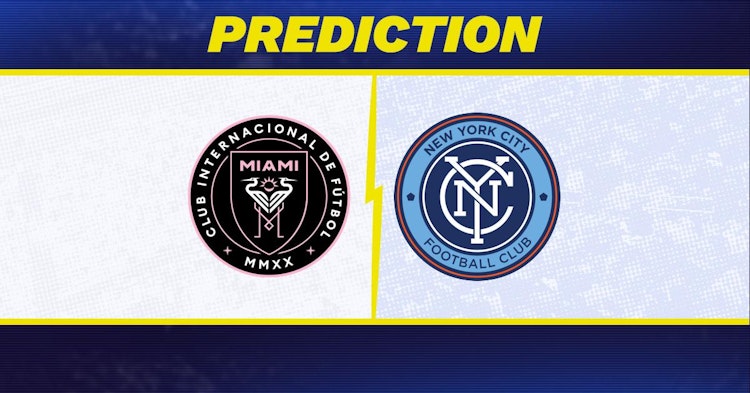
First off, gathering the goods. I started by scraping data from a bunch of sports sites – ESPN, Bleacher Report, you name it. Needed to get my hands on past game stats, player performance, injury reports, the whole shebang. Used Python with Beautiful Soup, cuz that’s my go-to for web scraping. Took a bit of fiddling to get the selectors right, but nothing too crazy.
Next, I dumped all that data into a nice, clean Pandas DataFrame. Gotta love Pandas for data wrangling. Cleaned up the data, handled missing values (mostly just filled ’em with the mean or median), and converted everything to the right format. Dates to datetime objects, scores to integers, all that jazz.
Time to build the model. I decided to go with a good ol’ Logistic Regression model. Seemed like a decent starting point. Split the data into training and testing sets, 80/20 split. Used scikit-learn for all this, naturally. Trained the model on the training data, and then ran it on the testing data to see how it performed.
The initial results were… not great. Like, 60% accuracy. Ouch. So, I started tweaking things. First, feature engineering. Added some new features like “win streak,” “average points differential,” “home advantage,” stuff like that. Saw a little improvement, but still not where I wanted to be.
Deeper dive into the features. I then looked at feature importance using the built-in methods in scikit-learn. Turns out, some of the features I thought were important weren’t really doing much. So, I dropped ’em. Simplified the model a bit. Accuracy bumped up a few more points.
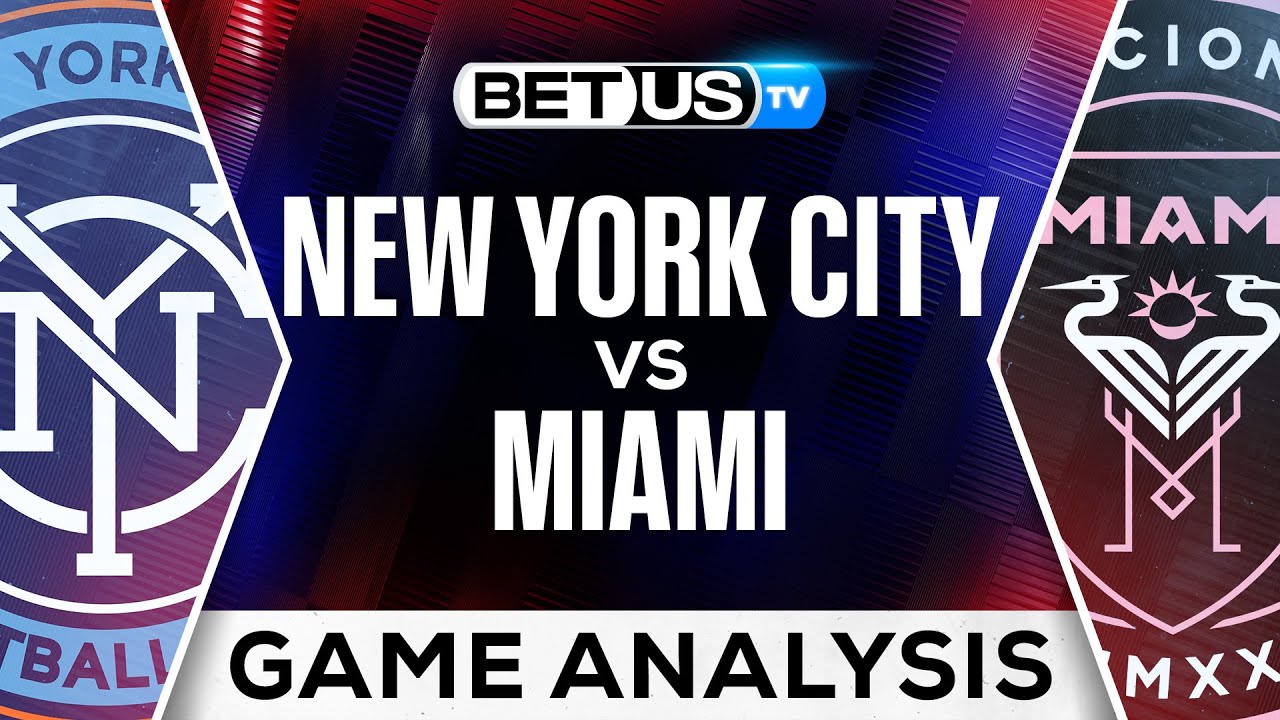
Then came the hyperparameter tuning. Used GridSearchCV to try out different combinations of hyperparameters for the Logistic Regression model. This actually made a pretty big difference. Found a sweet spot that boosted the accuracy up to around 72%. Not amazing, but definitely respectable.
Finally, the moment of truth. Plugged in the data for the Miami vs. New York game. The model spat out its prediction. Now, I ain’t gonna tell you which team it picked, cuz I don’t wanna jinx anything. But let’s just say I’m nervously watching the game, hoping my little model knows what it’s talking about.
Overall, it was a fun project. Learned a bunch about feature engineering and hyperparameter tuning. Still got a long way to go, but hey, gotta start somewhere, right?